When artificial intelligence takes the wheel
Several SDSU computer scientists and engineers do not fully trust self-driving vehicles quite yet but they are hopeful that, thanks to their own research, they might soon be able to let computers take the wheel.
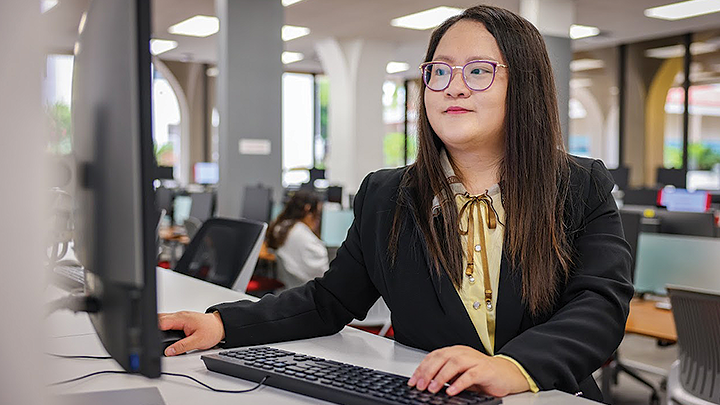
Originally published in Engage & Innovate - SDSU's Research, Scholarship and Creative Activities Magazine
California’s roads are some of the worst in the nation. Potholes, bumps and cracks are ever-present despite the state government budget spending more than $3 billion on streets and roads in 2022-2023.
“Traditionally, identifying pavement problems is done manually by professionals, and this requires a large amount of labor and extensive domain knowledge,” said Vivian Huangfu, a management information systems professor and the director of SDSU’s AI4 Business lab. “Given the complex and vast network of roadways it is almost impossible to inspect them all manually.”
Automating detection of pavement hazards is therefore essential to save time and taxpayer money.
Huangfu, together with a team of researchers including SDSU undergraduates, applied artificial intelligence (AI) to the costly problem of identifying potential road hazards in need of fixing.
The team divided high-resolution images of pavement into smaller patches, then fed these images into an AI algorithm which combined them into a large-scale image of a stretch of pavement.
With these larger composite images, another machine learning algorithm could extract details about cracks and flaws. This algorithm was able to classify images into different types of hazards, easing engineers’ burden of deciding which problem areas to prioritize.
Modeling Materials
With funding from SDSU’s Division of Research and Innovation, Sara Adibi, a mechanical engineer, is using machine learning-based models to enhance the design of damage-resistant materials.
“By making extremely precise adjustments at the atomic level, we can achieve different material properties, effectively tailoring them to our specific needs,” she said.
Applying the laws of physics to computer-generated data, Adibi and her students are simulating what would happen if they make small changes in the ratio or position of steel, aluminum and titanium atoms within a material.
These physics-informed computational models can predict how adding pores or some form of reinforcement would enhance a material’s strength or ability to withstand sudden impact.
Adibi says these models save the years and thousands of dollars it would take to run experiments, accelerating the development of potentially indestructible cars and protective gear.
Eyes in the Sky
Huangfu and her collaborators also developed technology that can follow a single car or truck as it appears in traffic camera feeds around a city.
The researchers accomplished this by analyzing the brightness and color of pixels in traffic camera footage to outline features of individual vehicles and assign each feature a level of importance.
The team next used a second set of artificial intelligence algorithms, called a convolutional neural network, to match identical vehicles across snapshots.
Prioritizing features based on their uniqueness reduced the time taken to visually match vehicles and was more accurate than other algorithms, said Huangfu. “This process eliminates time and cost needed to visually match vehicles and increases identification accuracy.”
This computer vision algorithm has the potential to streamline traffic patterns and assist in criminal investigations that require information about vehicle locations.
Without license plate information, machine learning algorithms identified distinctive features of individual cars. (Credit: Rong Qin, Vivian Huangfu, Devon Hood, James Ma, Sheng Huang)
Autonomous Automobiles
Self-driving cars also need to recognize objects like traffic signs and other vehicles, but have the added challenge of needing to perform calculations in fractions of seconds to avoid collisions.
To enable such quick responses, SDSU researchers are optimizing how the computers within self-driving vehicles allocate their finite resources to their cameras, sensors, and operating systems. They are also adapting these algorithmic decisions to perform equally well in cold weather and on dangerous roads.
Self-driving cars must simultaneously perform multiple calculations to quickly detect obstacles and decide how to avoid them. (Photo by Xiaobai Liu)